Kubernetes Deployment Strategy: Common Mistakes to Avoid
In Kubernetes deployments, certain missteps happen frequently. We have asked our in-house DevOps specialist, Mikhail Shayunov, about these typical blunders and their origins. Also, Mikhail has covered possible effective solutions and preventative measures to update your Kubernetes deployment strategy. Delve in and see how you can make deployment a more streamlined process in your company.
What’s the biggest mistake Kubernetes developers make?
In the Kubernetes deployment strategy, insufficient attention is often paid to resource planning. Sometimes, I encounter clusters in which developers have yet to describe the requirements for limits and requests, have not configured horizontal pod autoscaling, or have not correctly calculated the capacities of worker nodes. All this leads to hard-to-diagnose application issues. The reverse side of this problem is that developers request many times more capacities than necessary, which does not negatively affect performance but dramatically increases the difference in the cost of the solution compared to the classic architecture.
Another significant and often overlooked error in Kubernetes development is the failure to specify CPU and memory usage limits and requests. Requests refer to the minimum resources required for an application development, while limits define the maximum resources a container can utilize. Not setting these limits can overload worker nodes, resulting in poor application performance. Conversely, setting too low limits can lead to CPU underperformance and OOMkill errors. Both cases result in Kubernetes becoming ineffective.
A lack of resource control also means the application is not adequately monitored, making it challenging to identify and resolve potential issues or bottlenecks.
What can be a game-changer if the company wishes to update its Kubernetes deployment strategy?
Consider the following rules of thumb to avoid typical pitfalls with Kubernetes.
Create a test environment to check application launches
Define correct performance parameters, allocate the right amount of CPU and memory, and define metrics for usage in Horizontal Pod Autoscaler (HPA) for resource management and scalability.
Remember to put the correct settings of node pool autoscaling on the cloud side. For instance, HPA is usually implemented as a deployment entity within a cluster. It automatically adjusts the quantity of Kubernetes nodes in your cluster according to your needs. If the number of pending pods increases, indicating resources in the cluster are inadequate, the cluster autoscaler automatically includes additional nodes.
Optimize resource allocation and scalability in Kubernetes
Effective workload management is crucial to prevent burnout and ensure successful project execution, especially for remote development teams. It requires understanding each team member’s strengths, weaknesses, and current capacity. To accurately estimate team workload, it’s crucial to establish initial task estimates, track remaining work for each task, and maintain a regular work log where team members record their work hours. This helps identify who is overloaded or underloaded, allowing you to redistribute tasks and balance the workload.
Conduct stress testing and automated tests
Stress testing on your application allows you to identify the threshold at which the system or software fails. By observing the system’s reactions in different scenarios, you can establish bottlenecks in the defined autoscaling policies and dedicate resources as requests and limits, ensuring consistent service and optimal performance.
Need DevOps services ?
What’s the best way to avoid future mistakes?
When starting, never allow manual changes to infrastructure or configurations.
For infrastructure, use Kubernetes deployment tools, for instance, infrastructure-as-code (IaC), and for deploying components within Kubernetes, use templating tools such as Helm or Kustomize. Additionally, applying thinking-forward thinking and using DevSecOps tools might help you significantly in avoiding possible pitfalls related with security.
Set up repositories for this code in any version control system you are comfortable with and CI/CD pipelines for automatic changes. In general, this practice will help identify the causes of bugs and address them more effectively.
In the long run, Kubernetes is not an orchestration tool to be afraid of. It will bolster you with a different level of confidence, beating other technologies by a mile. The flexibility and stability it provides will significantly enhance your overall application performance.
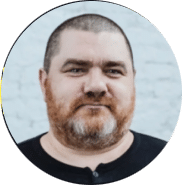
Mikhail Shayunov
Head of DevOps, has 17+ years of experience in system administration and security infrastructure development and 10+ years of in-depth experience designing, implementing, and scaling highly efficient technical environments for banking IT systems and technologies.