IoT & ML-based Energy Management Solution
IOT FOR ENERGY MANAGEMENT
IoT & ML-Based Energy Management Solution
The team developed software for IoT energy management, specifically tailored to monitor wind turbines and manage energy production.
#IoT
#Energy
#ML
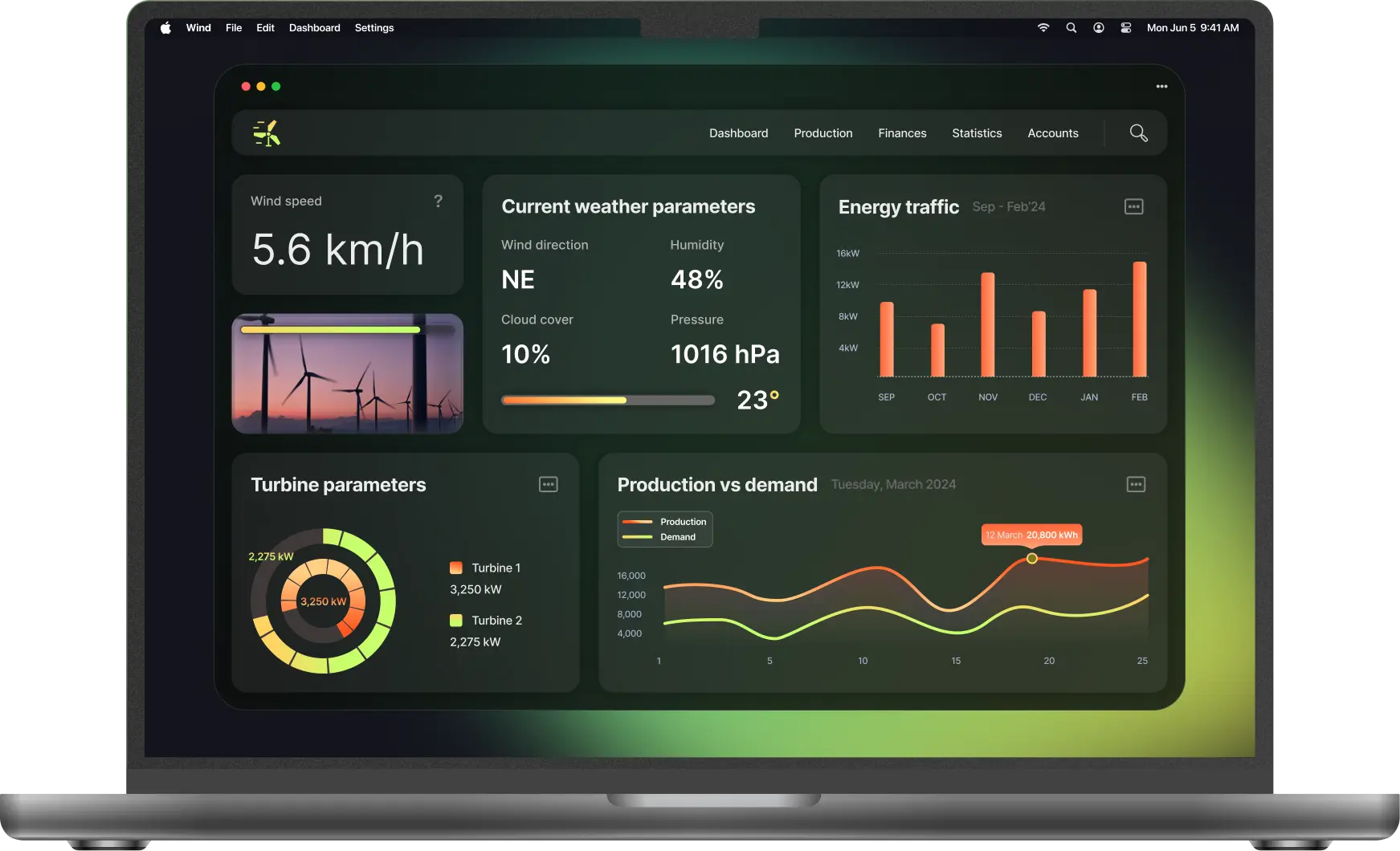
Client*
A leading business in the renewable energy industry for over 20 years, specializing in wind energy and overseeing a vast network of wind turbines across multiple regions.
*We cannot provide any information about the client or specifics of the case study due to non-disclosure agreement (NDA) restrictions.
Project in numbers
duration
Ongoing project
14 specialists
The team involved in the project
industry
Energy
solution
Data analytics
JavaScript, React, Redux, Python, FastAPI, Apache Spark, Kubernetes, Docker, AWS, PostgreSQL, Grafana
Services
1 x Business analyst
1 x Project manager
1 x Solution architect
3 x Back-end developers
1 x Front-end developer
1 x Embedded developer
1 x ML developer
1 x DE developer
1 x DevOps Specialist
2 x QA engineers
1 x Stakeholder’s SME
Challenge
Build an IoT energy management solution empowered with ML algorithms for real-time monitoring and predictive analysis of wind turbine performance. The main goal is to prevent system malfunctions that could cause power outages and costly repairs.
Solution & functionality
The team came up with an IoT & ML-driven energy management software solution that predicts energy production. An advanced platform provides real-time updates on the status of each wind turbine based on the information accumulated from meteorological sensors and turbines.
Programmable logic controllers (PLC)
We utilized programmable logic controllers (PLCs) to gather data from sensors placed across the wind turbines. They monitor various operational metrics, like wind speed, turbine rotation speed, temperature, vibration, and torque, process the data and provide a precise overview of the wind turbine’s current performance, identify faults, and energy production efficiency. Additionally, system detects deviations, like an unexpected temperature rise or increased vibration — to prevent damage, it triggers alarms or shuts down the turbine. Such timely maintenance and malfunction prevention ensures balanced energy production and extends equipment lifespan.
Data visualization
To visualize data, our project team chose Grafana dashboards. We created customized actionable charts for IoT energy management displaying data like daily power output, turbine locations, weather patterns, and predicting future trends. Thanks to these visualizations operational managers have access to a real-time overview of turbine performance, while maintenance teams can quickly address turbine issues.
Data lake
The client needed a robust data lake, as they operate wind turbines across various regions. Our developers created a central repository to collect and store data from all turbines, regardless of their location, including structured, unstructured, and semi-structured data such as logs, sensor readings, and images. Data is collected from the PLCs and then stored and processed using AWS IoT Core and Lambda functions. Large datasets can be processed simultaneously, which greatly supports predictive maintenance and accelerates analysis and reporting.
Error prediction
Leveraging data science and MLOps, we developed a predictive model that evaluates various factors influencing turbine health, such as vibration and temperature levels, and performance metrics. This model continually learns from incoming data and enables the operational managers to detect warning signs of failures early. Upon identifying them, the energy management control system sends alerts to the maintenance teams so that they proactively address the issues before they cause breakdowns.
Analytical reports
The energy management system can generate analytical reports based on the historical and real-time data to provide insights into wind turbine performance. This data helps identify well-operating turbines and those needing maintenance. Also, by analyzing performance trends and external factors like weather, the system suggests ways to optimize energy consumption, determine ideal times for energy harvesting, manage storage, reduce costs, and streamline maintenance.
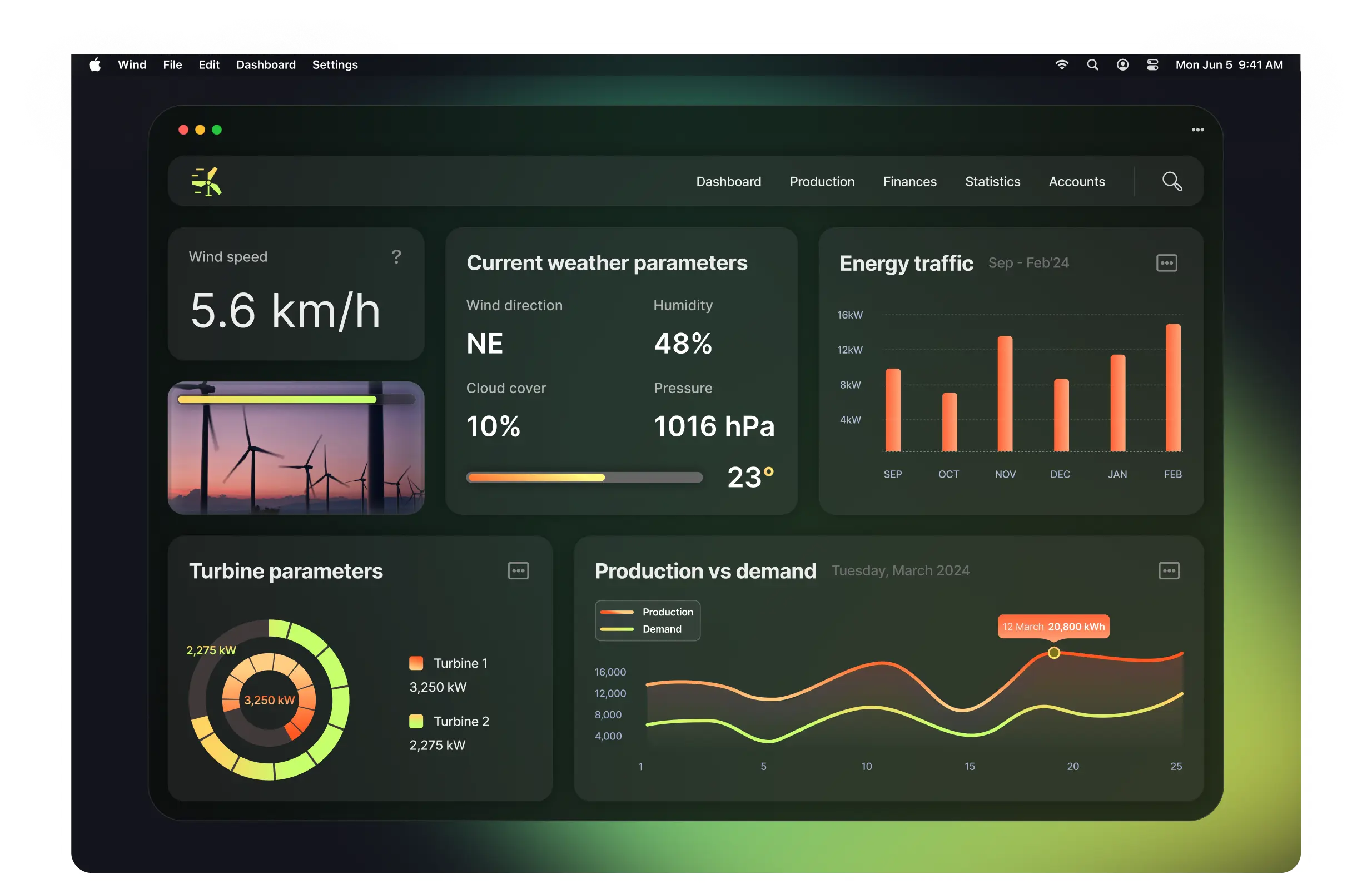
Results and business value
The team successfully implemented IoT in energy management, providing the client with a scalable energy management control system.
up to 6%
increase in energy production
18%
reduction in maintenance and repair costs
26
critical threats prevented
Benefits for client
The solution helps prevent system malfunctions that could cause power outages and costly repairs. As a result, the client achieved 18% reduction in maintenance and repair costs and up to 6% increase in energy production.
Related cases
Need assistance with a software project?
Whether you're looking for expert developers or a full-service development solution, we're here to help. Get in touch!
What happens next?
An expert contacts you after thoroughly reviewing your requirements.
If necessary, we provide you with a Non-Disclosure Agreement (NDA) and initiate the Discovery phase, ensuring maximum confidentiality and alignment on project objectives.
We provide a project proposal, including estimates, scope analysis, CVs, and more.
Meet our experts!
Viktoryia Markevich
Relationship manager
Samuel Krendel
Head of partnerships