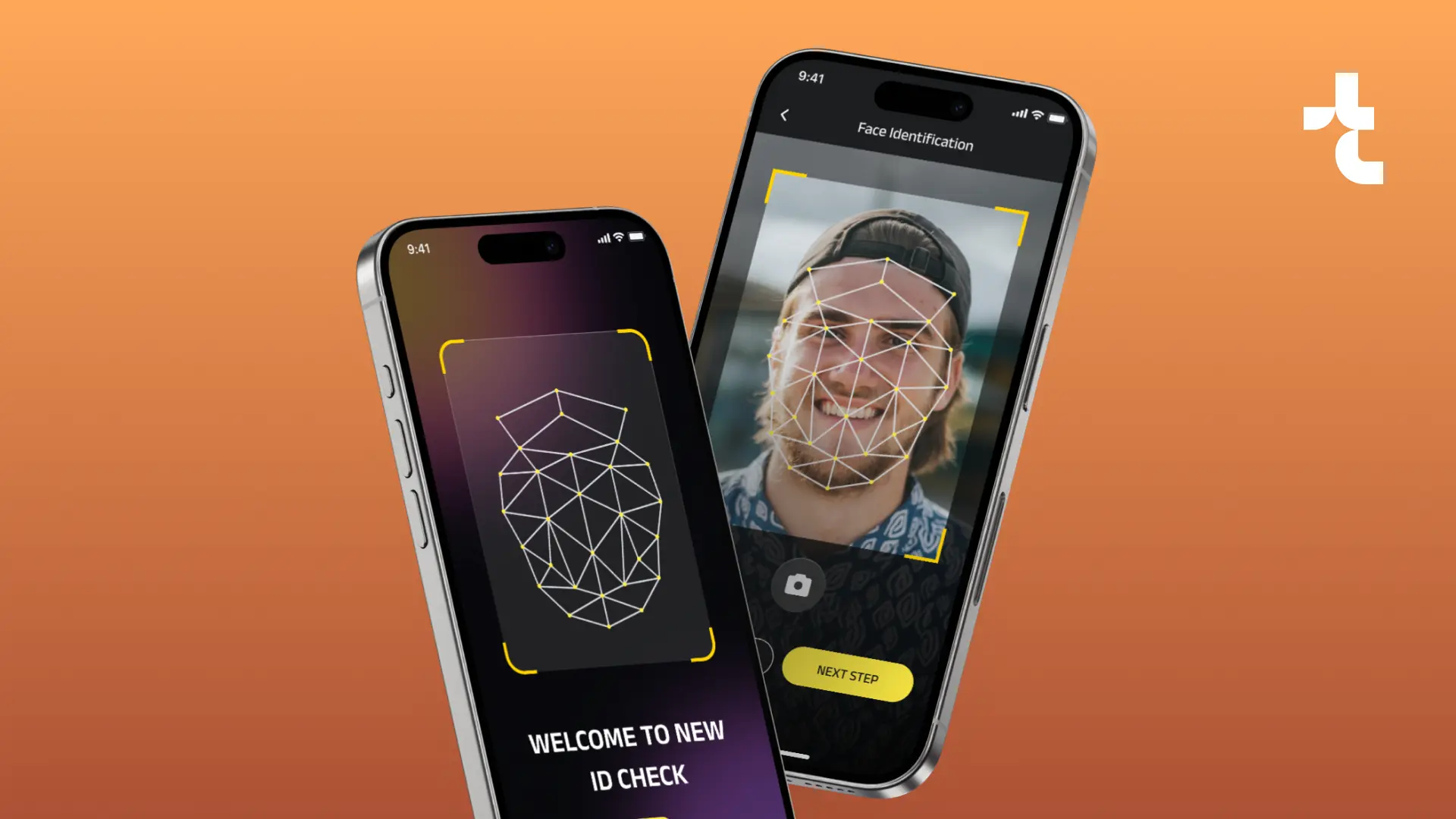
Python, Scala, DVC, MLFlow, Comet, Apache Spark MLLib, Scikit-learn, LightGBM, XGBoost, Hyperopt, PySpark, Numpy, Pandas, Scipy, Docker, Docker Compose, Kubernetes, Jenkins
Whether you're looking for expert developers or a full-service development solution, we're here to help. Get in touch!
An expert contacts you after thoroughly reviewing your requirements.
If necessary, we provide you with a Non-Disclosure Agreement (NDA) and initiate the Discovery phase, ensuring maximum confidentiality and alignment on project objectives.
We provide a project proposal, including estimates, scope analysis, CVs, and more.
Viktoryia Markevich
Relationship manager
Samuel Krendel
Head of partnerships