ML in banking: 5 times less fraud risk by spotting weird transactions
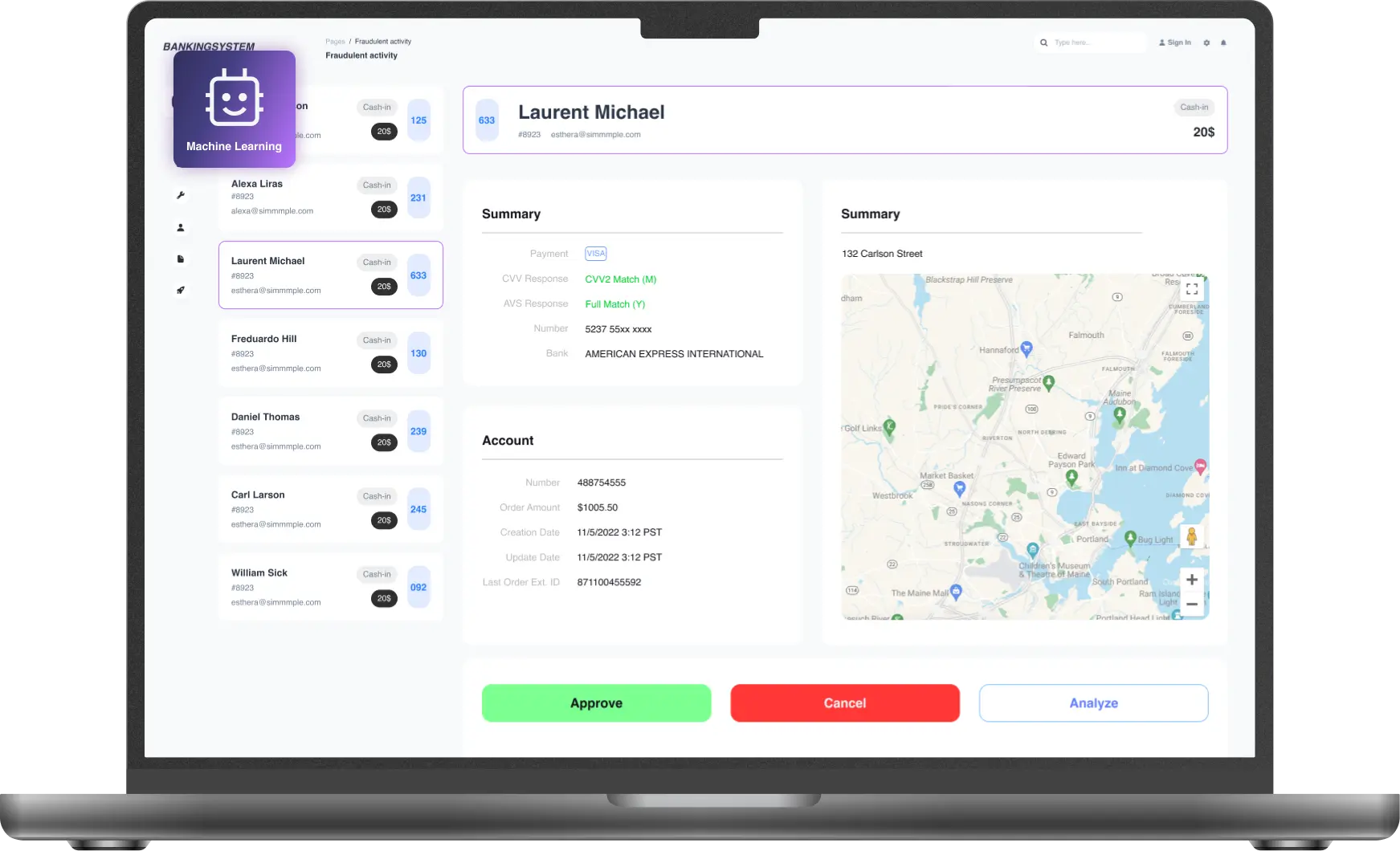
Client*
Project in numbers
Team involved in the project
Python, Scala, DVC, MLFlow, Comet, Apache Spark MLLib, Scikit-learn, LightGBM, XGBoost, Hyperopt, PySpark, Numpy, Pandas, Scipy, Docker, Docker Compose, Kubernetes, Jenkins
Challenge
Related objectives
Solution & functionality
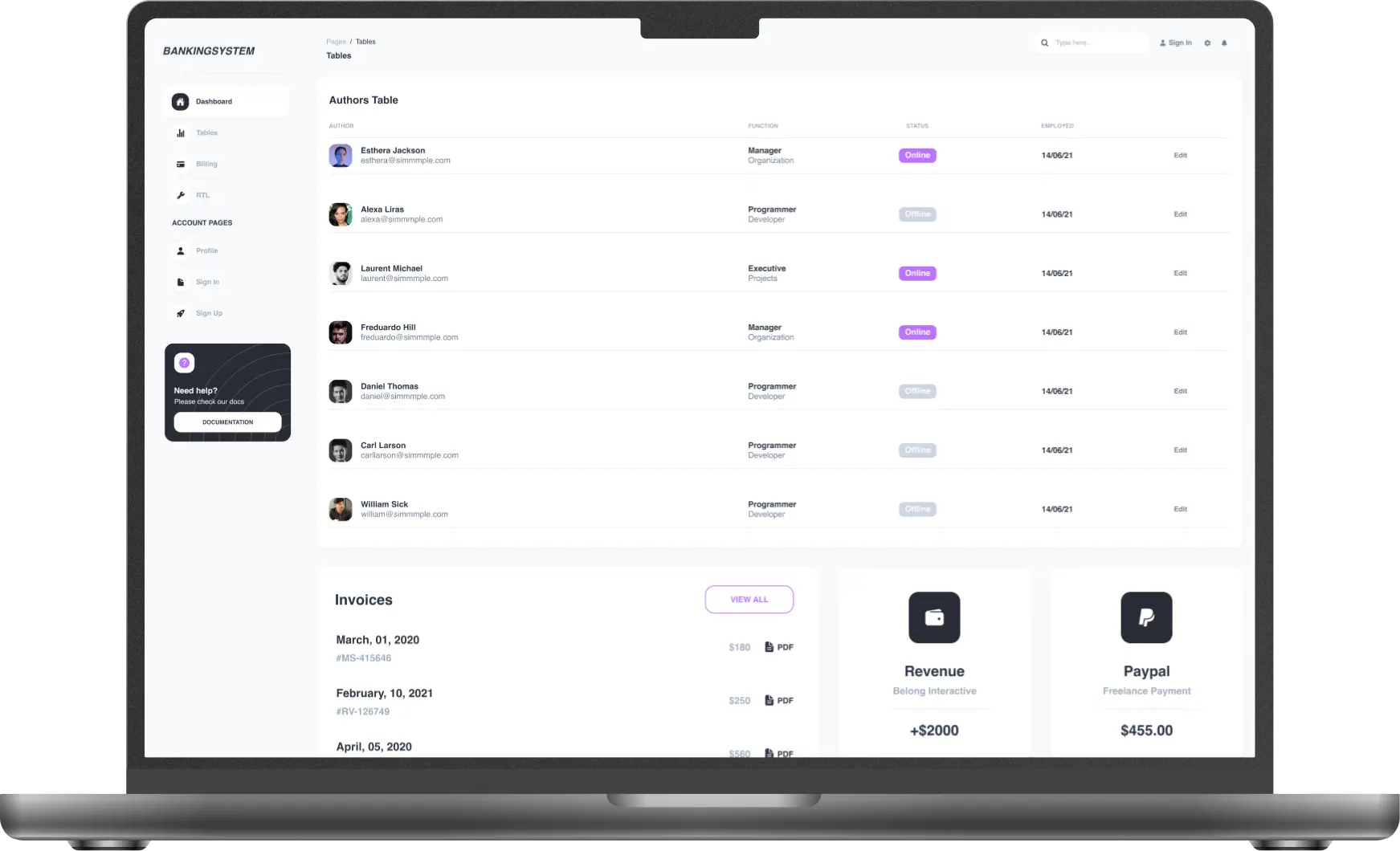
Aggregating data
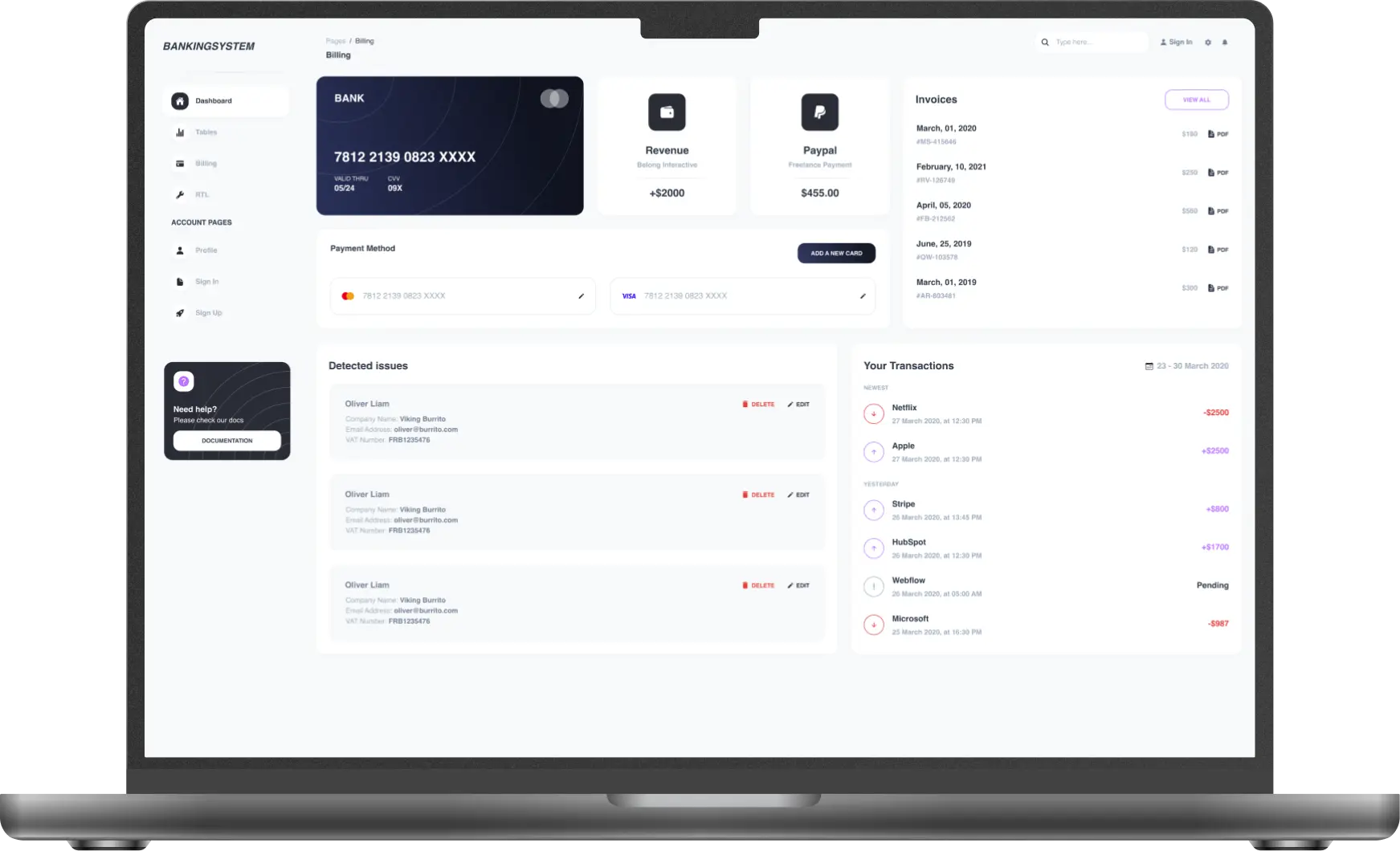
Detecting anomalies
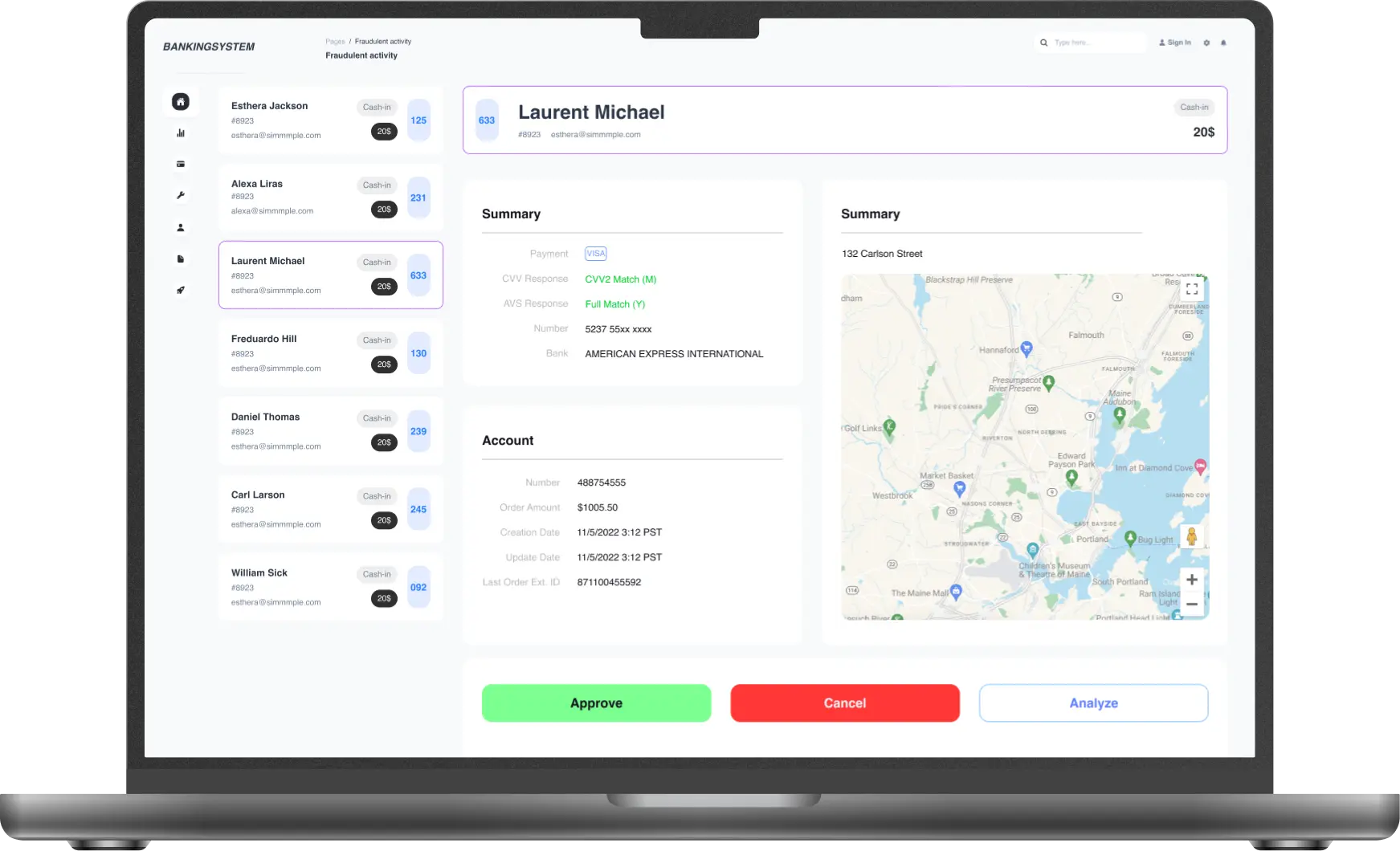
Training the ML model
Implementing the ML model
- If fraud odds are below 5%, the transaction gets the green light.
- If the odds range between 6% and 70%, an extra check like an SMS code, fingerprint, or secret question is needed.
- If the fraud chance tops 80%, the transaction’s axed, needing hands-on analysis.